Data Structure Essay代写:降维
Keywords:Data Structure Essay代写
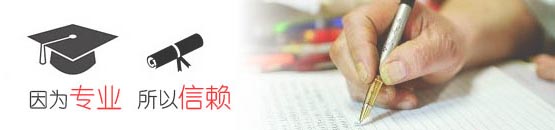
提出了一种统一的半监督降维流形学习框架。降维的目的是减少输入数据的复杂性,同时保留一些所需的数据的内在信息。(刘,等。2009)提出了一种基于动态互信息的特征选择方法。特征选择在数据挖掘和模式识别中起着重要的作用,特别是对大规模数据。由于数据挖掘能够识别新的,潜在的和有用的信息从数据集,它已被广泛应用在许多领域,如决策支持,模式识别和财务预测。特征选择是根据判别能力选择原始特征空间的子集来提高数据质量的过程。特征约简是指用于减少描述数据的维数的方法的研究。它的一般目的是采用较少的功能来代表数据和降低计算成本,而不会恶化辨别能力。(Upadhyay,et al。2013)提出了一个关于各种数据流程序和各种降维技术的比较分析的论文。在这项研究中,各种数据流挖掘技术和降维技术进行了评估的基础上,它们的使用,应用参数和工作机制。(shlens 2005)提出了一种基于主成分分析(PCA)教程。PCA被称为应用线性代数的最有价值的结果之一。PCA的目标是计算最有意义的基础,重新表达嘈杂的数据集。(Hoque,et al。2009)提出了一种用于蛋白质结构预测的扩展HP模型。本文提出了一个基于格的HP详细调查(疏水–亲水)从头蛋白质结构预测(PSP)。(Borgwardt,et al。2005)推荐一篇关于通过图形内核预测蛋白质功能的论文。蛋白质功能预测的计算方法--通过寻找相似序列的蛋白质推断蛋白质功能。模拟分子和原子机制定义的蛋白质的功能是超越目前的生物化学知识和可用的计算能力的能力。
Data Structure Essay代写:降维
proposed a unified semi supervised dimensionality reduction framework for manifold learning. The goal of dimensionality reduction is to diminish complexity of input data while some desired intrinsic information of the data is preserved. (Liu, et al. 2009) proposed a paper about feature selection with dynamic mutual information. Feature selection plays an important role in data mining and pattern recognition, especially for large scale data. Since data mining is capable of identifying new, potential and useful information from datasets, it has been widely used in many areas, such as decision support, pattern recognition and financial forecasts. Feature selection is the process of choosing a subset of the original feature spaces according to discrimination capability to improve the quality of data. Feature reduction refers to the study of methods for reducing the number of dimensions describing data. Its general purpose is to employ fewer features to represent data and reduce computational cost, without deteriorating discriminative capability. (Upadhyay, et al. 2013) proposed a paper about the comparative analysis of various data stream procedures and various dimension reduction techniques. In this research, various data stream mining techniques and dimension reduction techniques have been evaluated on the basis of their usage, application parameters and working mechanism. (Shlens 2005) proposed a tutorial on Principal Component Analysis (PCA). PCA has been called one of the most valuable results from applied linear algebra. The goal of PCA is to compute the most meaningful basis to re-express a noisy data set. (Hoque, et al. 2009) proposed an extended HP model for protein structure prediction. This paper proposed a detailed investigation of a lattice-based HP (Hydrophobic – Hydrophilic) model for ab initio Protein Structure Prediction (PSP). (Borgwardt, et al. 2005) recommended a paper about protein function prediction via graph kernels. Computational approaches to protein function prediction infer protein function by finding proteins with similar sequence. Simulating the molecular and atomic mechanisms that define the function of a protein is beyond the current knowledge of biochemistry and the capacity of available computational power.