美国加州洛杉矶代写:现象学方法
Keywords:美国加州洛杉矶代写:现象学方法
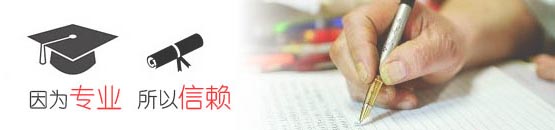
现象学方法假定第一人称熟悉研究者感兴趣的特定经验。数据分析本质上是对一种有意识的体验的描述,就像参与者所生活的一样,并且呈现给研究者,而研究者并不干涉。然后,研究者可能试图从他们特定的现象学观点来解释经验。例如,诠释学现象学家,如海德格尔,将试图通过将体验置于社会和语言语境中来理解它(例如,谁参与其中,以及双方如何交流)。相比之下,自然主义构成现象学家将把经验与自然联系起来,寻找与自然环境(如气候、文化、生态)的联系。无论他们的现象学领域是什么,研究者都有必要分析所呈现的经验类型,找出任何独特的特征以进行进一步的研究。最近,数据分析可能需要一种逻辑-语义方法,目的是确定一种经历的真实性(例如,“这种疾病可以治愈”)和满足一种意图所必需的条件(例如,“如果我服药,我会感觉更好”)。现象学家也使用现代技术来分析定性数据,如专题分析、类型学、引文等等。实验中的数据分析需要使用统计检验,以便在操纵自变量之后,确定因变量中任何观察到的变化的“显著性”。通常,一个“显著性水平”被设定,描述一个特定的概率(例如。05),观察组之间的差异或偶然发生的情况。通常情况下,概率必须等于或小于选择的显著性水平,才能将测试结果视为显著性。如果不使用统计工具,实验者就不会试图对数据“强加”任何解释或主观分析,因为统计工具引入了一些数学客观性。然而,获得显著结果的可能性通常受到分析和方法考虑因素的影响,例如样本大小和所选统计检验的敏感性。此外,有统计学意义的结果可能没有或很少有临床意义,例如在高质量生命年、发病率和死亡率方面。
美国加州洛杉矶代写:现象学方法
The phenomenological method assumes first-person familiarity with the particular experience of interest to the researcher. Data analysis essentially entails description of a conscious experience exactly as it is lived by the participant and presented to the researcher, who does not interfere. The researcher may then attempt to interpret the experience from their particular phenomenological perspective. For example, hermeneutical phenomenologists, such as Heidegger, will try to make sense of the experience by placing it in a social and linguistic context (e.g. who else is involved, and how do the parties communicate). By contrast a naturalistic constitutive phenomenologist will relate the experience to nature, seeking out links with natural environment (e.g. climate, culture, ecology). Regardless of their area of phenomenology, it is essential for the researcher to analyse the type of experience presented, identifying any unique features for further investigation. More recently, data analysis may entail a logico-semantic approach that aims to identify the truth of an experience (e.g. “this disease can be cured”) and the conditions necessary to satisfy an intention (e.g. “I will feel better if I take my medicine”). Phenomenologists also use modern techniques for analysing qualitative data, such as thematic analysis, typologies, quotations, and so on. Data analysis in experimentation requires the use of statistical tests in order to establish the “significance” of any observed changes in the dependent variable, following manipulation of the independent variable. Usually, a ‘level of significance’ is set, depicting a specific probability (e.g. .05) that observed differences between groups or conditions occurred by chance. Typically, the probability of chance must be equal to or less than the chosen significance level in order for the test results to be regarded as significant. There is no attempt by the experimenter to “impose” any interpretation or subjective analysis on the data without the use of statistical tools, which introduce some mathematical objectivity. However, the likelihood of obtaining significant results is often affected by analytic and methodological considerations, such as the sample size and the sensitivity of the chosen statistical test. Furthermore, results that are statistically significant may nevertheless have little or no clinical significance, for example in terms of Quality Life Years, and morbidity and mortality rates.